AWS and Google (and Microsoft Azure) have services called IAM, which stands for Identity and Access Management. The IAM service serves roughly the same purpose in each provider: to authorize principals (users, groups, or service accounts) to access and use services and resources on the respective platform. There are subtle yet significant differences and distinctions across the major cloud providers.
This article will look at the differences between IAM in AWS and IAM in Google.
Firstly, Google's IAM is a slight misnomer regarding the I as it does not manage identities (with the single exception of service accounts). Google identities are sourced from Google accounts created and managed outside the Google Cloud Platform. Google identities (users and groups) are Google accounts which could be accounts in a Google Workspace domain, a Google Cloud Identity domain, or Gmail accounts. Still, these accounts are NOT created or managed using the Google IAM service.
Conversely, AWS IAM creates and manages identities for use in the AWS platform (IAM Users), which can be used to access AWS resources using the AWS console or programmatically using API keys.
It can be confusing for people coming from AWS to Google or vice-versa. Some of the same terms exist in both providers but mean different things. The table below summarises the difference in the meaning of terms in both providers. We will unpack this in more detail in the sections that follow.
* nearest equivalent
An AWS IAM Role is an identity that can be assumed by trusted entities using short-lived credentials (issued by the AWS Security Token Service or STS API). A trusted entity could be an IAM User, Group, or a service (such as Lambda or EC2).
Permissions are assigned to IAM Roles (and IAM Users and Groups) through the attachment of IAM Policies.
AWS Policies are collections of permissions in different services which can be used to Allow or Deny access (Effect); these can be scoped to a resource or have conditions attached. The following is an example of an AWS Policy:
{
"Version": "2012-10-17",
"Statement": [
{
"Effect": "Allow",
"Action": "ec2:Describe*",
"Resource": "*"
},
{
"Effect": "Allow",
"Action": "autoscaling:Describe*",
"Resource": "*"
}
]
}
Policies in AWS can be Managed Policies (created and managed by AWS) or Customer Managed Policies - where the customer defines and manages these policies.
An IAM Role that is used by a service such as Lambda or EC2 will have a Trust Policy attached, which will look something like this:
{
"Version": "2012-10-17",
"Statement": [
{
"Effect": "Allow",
"Principal": {
"Service": "lambda.amazonaws.com"
},
"Action": "sts:AssumeRole"
}
]
}
Roles in Google IAM are NOT identities ; they are collections of permissions (similar to Policies in AWS). Roles can be of the following types:
Basic Roles are coarse-grained permissions set at a Project level across all services, such as Owner, Editor, and Viewer. Support for Basic Roles is maintained by Google, however, Google does not recommend using Basic Roles after a Project is created.
Predefined Roles are pre-curated sets of permissions that align with a role that an actor (human or service account) would play, such as BigQuery Admin. Predefined roles are considered best practice in Google as the permissions for these roles are maintained by Google. Predefined Roles in Google would be the nearest equivalent to Managed Policies in AWS.
Custom Roles are user-specified and managed sets of permissions. These roles are scoped within your Project in Google and are your responsibility to maintain. Custom Roles are typically used when the permissions granted through a Predefined Role are too broad. Custom Roles would be the nearest equivalent of Customer Managed Policies in AWS.
Roles (collections of permissions) are attached to Principals (Identities such as users (Google accounts), groups and service accounts through IAM bindings. The example below shows a binding between a user principal (a Google Workspace account) and a predefined role (BigQuery Admin) within a GCP project:
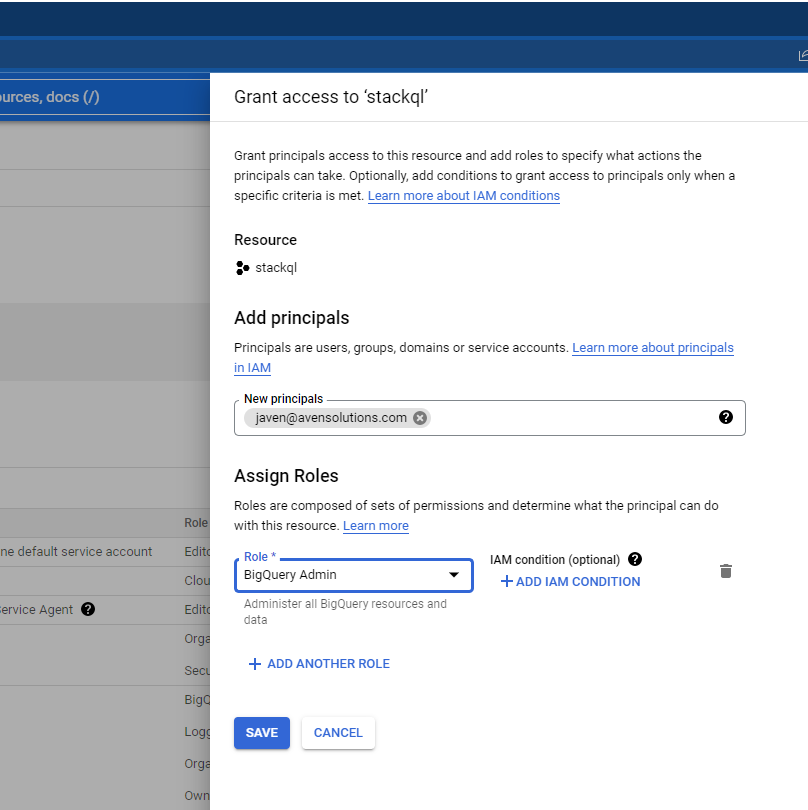
A Policy in Google is a collection of IAM Bindings between members (principals) and roles. An example policy would be:
{
"bindings": [
{
"members": [
"user:javen@avensolutions.com"
],
"role": "bigquery.admin"
},
... another binding ...
]
}
A Service Account in GCP is a password-less identity created in a GCP Project that can be used to access GCP resources (usually by a process or service). Service accounts are identified by an email address, but these are NOT Google accounts (like the accounts used for users or groups). Service accounts can be associated with services such as Compute Engine, Cloud Functions, or Cloud Run (in much the same way as AWS Roles can be assigned to services such as Lambda functions or EC2 instances). Google Service accounts can use keys created in the IAM service, which are exchanged for short-lived credentials, or service accounts can use get tokens directly, which include OAuth 2.0 access tokens and OpenID Connect ID tokens. Service accounts in Google are the nearest equivalent to AWS IAM Roles.
AWS (save AWS Organizations) is a flat structure with no inherent hierarchy and is oriented around regions that are seperate API endpoints (almost providers unto themselves); IAM, however, is a global service in AWS.
In contrast, GCP is hierarchical and globally scoped for all services, including IAM. Resources (such as Google Compute Engine Instances or Big Query Datasets) are created in Projects (similar to Resource Groups in Azure). Projects are nested under a resource hierarchy, starting at the root (the organization or org). Organizations can contain folders, which can be nested, and these folders can contain Projects.
IAM Bindings (and the permissions they enable) are inherited from ancestor nodes in the GCP hierarchy. A Principal's net effective permissions are the union of the permissions assigned through IAM Bindings in the Project and the permissions set through IAM Bindings in all ancestor nodes (including Folders and the Org itself).
IAM governs access and entitlements to services and resources in cloud providers, although the design, implementation, and terminology are quite different as you get into the details. This is not to say one approach is better than the other, but as a multi-cloud warrior, you should understand the differences.
if you have enjoyed this post, please consider buying me a coffee ☕ to help me keep writing!